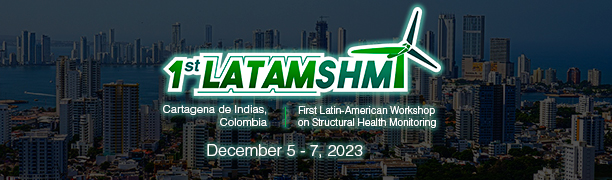
Damage Classification Utilizing Autoencoders and Convolutional Neural Networks
Please login to view abstract download link
Structural Health Monitoring (SHM) is a growing field in civil engineering and has relevance for detecting changes in the state of structures, including identifying damage conditions. SHM strategies commonly employ Artificial Intelligence (AI) techniques on raw dynamic data measured from structures to perform classifications or extract features from the original data. Among the AI algorithms for SHM, autoencoder, and convolutional neural networks have been identified as promising solutions, being the focus of this article. Both algorithms are applied to identify eight damage scenarios in a beam starting from the time histories of the tested structure, pointing out the advantages and disadvantages of each algorithm. The authors tested the beam through monitoring based on image processing using a high-speed camera. By comparing the results obtained from both algorithms, the researchers were able to highlight their respective strengths and weaknesses in the context of SHM. This information can assist engineers and researchers in selecting the most appropriate algorithm based on the specific requirements of their monitoring project.