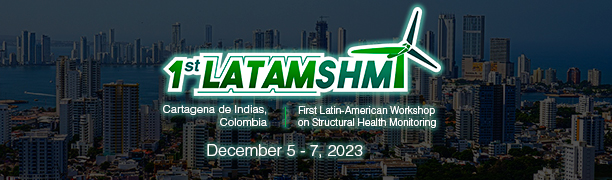
Value of Information Analysis Accounting for Sensor Data Quality: focus on drift
Please login to view abstract download link
Structural health monitoring plays a crucial role in assessing the condition of civil structures, providing information for regular maintenance and post-disaster emergency management. However, the reliability of structural health monitoring outcomes can be compromised by sensor malfunctions. Over the past two decades, sensor validation tools have been proposed to identify and discard abnormal measurements before extracting information from the structural health monitoring system. The long-term benefits of structural health monitoring systems are commonly evaluated without considering the possibility of faulty sensors. This can lead to suboptimal maintenance decisions. Recently, a Bayesian decision theory-based framework has been introduced to account for different data quality issues and quantify the benefit of implementing a sensor validation tool. This novel approach expands the traditional Value of Information concept to encompass multiple "functioning" states of the structural health monitoring system. This paper mainly focused on a specific data quality issue, i.e., bias or drift in the monitoring outcome. Previous applications of this framework regard simplified decision scenarios, where the monitoring system was either “damaged” or “undamaged”, considering a fixed drift value. In this paper, the impact of uncertain drift levels on the Value of Information in structural health monitoring is investigated, addressing real-world complexities. A numerical case study is considered to illustrate the practical implications of the VoI framework.