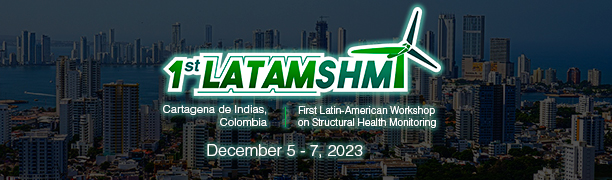
Advancing Wind Turbine Reliability: Machine Learning-Based Early Detection of Rotor Imbalance for Proactive Maintenance Strategies
Please login to view abstract download link
The early detection of rotor imbalance is crucial for maintaining the reliability and operational efficiency of wind turbines. In this study, we present a comprehensive methodology that leverages vibration-response-only accelerometer measurements and utilizes advanced machine learning techniques, specifically the extended isolation forest (EIF), for the early identification of rotor imbalance. The proposed methodology encompasses several key steps. Initially, accelerometer data is collected and processed to extract relevant features, including permutation entropy, fractal dimension, and kurtosis, which capture the characteristic patterns of the vibration signals associated with rotor imbalance. Subsequently, an EIF model is trained on the extracted features using data exclusively from healthy wind turbines. This model enables accurate anomaly detection and facilitates proactive maintenance actions. Experimental validation is conducted on a laboratory wind turbine subjected to controlled rotor imbalance, demonstrating the feasibility and effectiveness of the proposed methodology. The results showcase its capability to successfully detect and diagnose rotor imbalance faults, providing an early warning system for turbine operators. Such proactive approach empowers timely maintenance interventions, minimizing downtime, and reducing repair costs. The integration of machine learning techniques, specifically the EIF algorithm, with vibration-response data offers a data-driven approach for structural health monitoring of wind turbines. This approach addresses the main objective of detecting rotor imbalance and contributes to the advancement of predictive maintenance strategies in the wind energy industry. By harnessing the power of machine learning and utilizing vibration-response data, operators can implement proactive maintenance plans, thereby enhancing the reliability and performance of wind turbines while mitigating operational risks and financial losses.