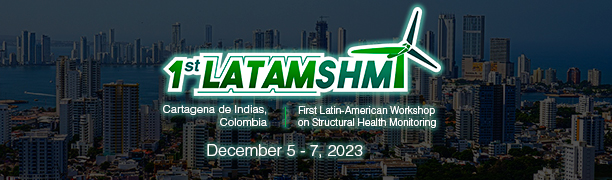
Structural health monitoring in a jacket-type wind turbine foundation: a minimum distortion embedding approach
Please login to view abstract download link
The extreme environmental conditions to which offshore wind turbine foundations are subjected make reliable monitoring methods necessary to predict possible structural damage. A data-driven approach was used to perform the structural health monitoring of a laboratory-scaled jacket-type wind turbine foundation. A white noise signal simulating the waves and wind in the sea was applied and amplified to the structure. The vibration-only response was measured by eight tri-axial accelerometers distributed in the structure. Five different structural classes, composed of the healthy and 4 unhealthy, were correctly classified using the following approach. 2460 measurements were acquired for the healthy structure and 820 by each one of the four unhealthy structures for 5740 measurements in total. The data was arranged using an unfolded procedure resulting in a two-dimensional matrix. This resulting matrix has a size of 5740 x 58008. This resulting data has a high dimensionality. Therefore, using the minimum distortion embedding (MDE) approach, a dimensionality reduction procedure transforms the original data into a low dimensional space with fewer features. The low dimensional representation given by different distortion functions was compared changing the repulsive and attractive penalties. The reduced feature matrix serves as input to a machine learning classifier. Several tree-based classifiers like decision trees, random forest and Adaboost were compared. A 5-fold cross validation procedure was executed to reduce the overfitting finally a set of classification performance measures were calculated. The developed structural damage classification methodology yields high classification accuracies.