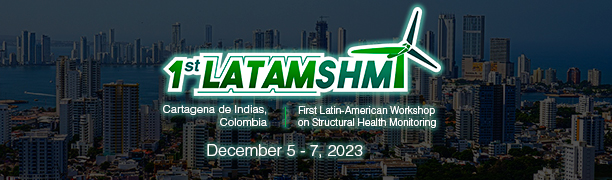
Temperature forecasting against sensors failures in an electric arc furnace
Please login to view abstract download link
Monitoring structural health in industrial equipment is vital to ensure worker safety, preservation, and operational efficiency. A robust and well-maintained structure minimizes risks, extends equipment lifespan, and prevents costly production disruptions. Additionally, proper structural health contributes to process stability, energy efficiency, and compliance with industrial regulations, ensuring a safe and efficient work environment. In the mining industry, highly complex equipment such as Electric Arc Furnaces (EAFs) are used for melting metals and other materials. These furnaces consist of casings, refractory walls, electrodes, and other key components that endure high temperatures and mechanical loads during the melting process. These furnaces have a robust sensor network that monitors and controls important variables in the melting process. Analytical techniques and artificial intelligence models have been developed to predict key variables, such as internal furnace temperature, based on other variables. However, issues can arise in information availability and prediction model performance due to possible sensor network failures. The objective of this study was to analyze the performance of a recurrent neural network model (GRU) used in predicting the temperature of an Electric Arc Furnace in the Colombian mining industry. Three cases of sensor network failures were studied in furnace monitoring: abrupt failure (sensor calibration issue), gradual failure (component wear), and noise-induced failure. A comparison was made between the model’s performance and the number of sensors with failures present, establishing the behavior and influence of these failures on temperature prediction. This study is important in the application field, as it showcases the outstanding performance of these new technologies in industrial use. It highlights the potential benefits and impact that these advancements could have on various industries.