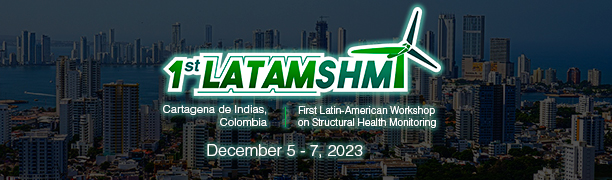
Forecasting of refractory wall thickness in an electric arc furnace used in ferro-nickel production
Please login to view abstract download link
The mining industry has overcome many challenges in recent years with the help of cutting-edge tech- nologies such as smart sensors, structural health monitoring, and AI-powered structural control, amongst others. These tools have revolutionized problem-solving with large amounts of data, allowing for au- tomation and online monitoring of large scale inraestructure, in this particular case eletric arc metal smelting furnaces. Among the multiple relevant elements, furnaces play a crucial role in the smelting process as they are responsible for melting down the raw materials and separating the metal from the im- purities, but operate in very harsh conditions, making its monitoring and safe operation a difficult task. Without furnaces, it would be impossible to extract metals like ferronickel, iron, copper, and aluminum from their ores. The temperature and conditions inside the furnace must be carefully controlled to ensure that the metal is of the desired quality and purity. Furnaces are made of refractory materials that can withstand high temperatures; however, these furnaces face significant challenges, including abrasive materials, high radiation levels, and high temperatures, leading to wear and tear on the refractory materials, which in turn may induce accidents that may be catastrophic. To prevent such incidents, it is essential to monitor the thickness of the refractory walls and the temperature in the furnace’s thermocouples; however, to have a direct measurement of the thickness of the refractory wall is not easy work because the operational conditions inside of the furnace do not allow for instrumentation. By developing and validating a thickness prediction model based on historical data and Machine learning techniques, we can accurately monitor a furnace’s state for up to 11 days from initial operating conditions, allowing for additional insight that may significantly extend the furnace’s operating window. The model was created using data from a furnace from Cerromato S.A., a ferronickel producer in the north of Colombia, which is one of the world’s biggest producers of ferronickel.