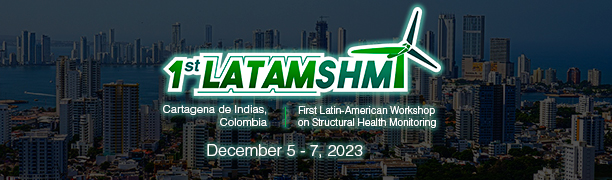
Comparative assessment of data normalization methods for modal-based SHM
Please login to view abstract download link
Accurate identification and assessment of damage in civil structures are crucial for ensuring structural integrity and public safety. Damage detection is one of the main aims of Structural Health Monitoring (SHM), which involves observing damage-sensitive features, such as natural frequencies, over time. However, those are typically sensitive not only to structural damage but also to environmental and operational variables (EOVs), such as humidity, operational loading, wind, and temperature. EOV can cause changes in natural frequency that are greater than those caused by actual damage, thus jeopardizing the reliability of automated damage detection by SHM systems. To address this challenge, several data normalization methods to be applied in the context of modal-based SHM have been proposed. These methods aim to mitigate the EOV effects, thereby allowing for more accurate and dependable damage identification. This research presents a comparative study of four methods: Multiple Linear Regression (MLR), linear Principal Component Analysis (PCA), Second-Order Blind Identification (SOBI), and Kernel Linear Principal Component Analysis (kPCA), evaluating their effectiveness using real-case data from the Z24 Bridge benchmark. Within the challenging context of the Z24 bridge, where EOVs induced a nonlinear behaviour, the potential benefits of incorporating complementary techniques, such as clustering, to augment the efficacy of traditional methods are discussed.