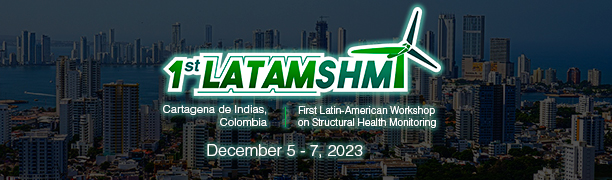
Fault detection in industrial pumps based on recurrent autoencoder neural networks
Please login to view abstract download link
The industry faces constant pressure to meet production targets and reduce costs, leading to overloaded physical assets and potential risks to safety, increased maintenance costs, and productivity losses. Maintenance plays a vital role in optimizing asset utilization. However, current preventive maintenance approaches often result in unnecessary inspections and part replacements, while still leaving room for unexpected failures. To address these challenges, it is becoming increasingly crucial to anticipate equipment failures and plan maintenance actions accordingly. With the widespread adoption of online monitoring systems, a wealth of data is generated, presenting an excellent opportunity to develop predictive maintenance techniques leveraging the Internet of Things (IoT) and Artificial Intelligence (AI). By harnessing these technologies, organizations can proactively identify potential issues, avoid catastrophic failures, and reduce the overall impact of maintenance activities on operations. In this study, an advanced system is developed to detect failures in water pumps within a mining desalination plant. The system utilizes Autoencoder Neural Networks, specifically incorporating recurrent layers that are commonly used for analyzing time series data. The monitored variables include current, flow, vibration, and temperature of both the pumps and electric motor, extracted from historical data. The implemented algorithms are trained to recognize normal operating patterns and identify anomalies associated with potential failures. To quantify the proximity of data to normal behavior, a normalized metric ranging from 0 to 1 is employed. A value closer to 0 indicates a lower level of danger, while a value closer to 1 indicates a higher likelihood of failure. The outcome of this research is a real-time early warning system that provides ongoing health monitoring of the equipment. During the validation process, the system successfully detected anomalies in the operation of the impulsion pumps and electric motor. Importantly, it demonstrated the ability to anticipate catastrophic failures within a timeframe of 5 to 72 hours. Furthermore, the system provides insight into the specific sensors that are most affected by the detected abnormalities, enabling targeted maintenance actions.