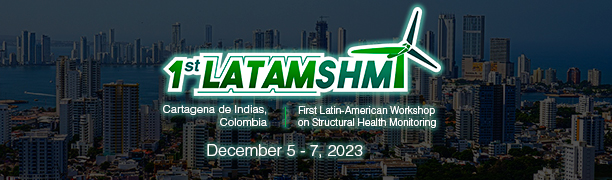
Advancing Wind Turbine Safety: Vibration-Based Early Detection of Blade Ice Accumulation Using Extended Isolation Forest
Please login to view abstract download link
The rapid growth in the installation of wind turbines has raised concerns about their safety and reliability. In response to this, structural health monitoring (SHM) and damage detection in wind turbines have become priority areas of research. This study focuses on developing an early detection method for ice accumulation on wind turbine blades, as ice poses substantial challenges to their structural integrity and aerodynamic performance. A novel methodology is proposed that takes advantage of vibration analysis and the advanced extended isolation forest (EIF) technique for ice accumulation detection. The proposed methodology is based on vibration analysis and the use of triaxial accelerometers to detect ice accumulation on the blades. The methodology stands out for its ability to utilize normal operating data from turbines, which constitute the majority of the available dataset. The damage detection process is conducted in three stages: 1) collection and processing of accelerometer data to extract relevant features, 2) training an unsupervised learning model, specifically the EIF algorithm, using data exclusively from turbines in healthy condition, and 3) evaluation and testing to detect blade ice accumulation through experiments with simulated additional masses. The results demonstrate the efficacy of the proposed methodology in successfully detecting and diagnosing ice accumulation, providing an early warning system for wind turbine operators. The application of the EIF technique enhances the accuracy and reliability of ice detection, enabling timely activation of blade de-icing processes. This proactive approach minimizes downtime, reduces repair costs, and mitigates the risk associated with ice detachment. By integrating the extended isolation forest technique with vibration-based analysis, this study contributes to the advancement of structural health monitoring in wind turbines. The methodology offers a robust and data-driven solution for early ice accumulation detection, facilitating improved operational safety and performance. Such proactive strategies empower wind turbine operators with the tools needed to mitigate risks, optimize maintenance plans, and ensure the sustained reliability of wind energy systems.